In this study, we will discuss how retail major Lenskart has benefitted from its partnership with GeoIQ, a location-based intelligence provider.
Having access to location-based data is immensely helping businesses that are running their decision-making engines on data. It has been established fairly well that data is the new fuel for businesses to improve their ROIs, reduce costs, and have better engagement with users and customers among other benefits. Talking specifically about the retail sector, location-based data and intelligence is a game-changer, especially for their expansion plans.
Location-based data and intelligence as a solution is in a very nascent stage. There could be a plethora of use cases for the same depending on business requirements that have not been thought of yet. Moreover, the technology behind these solutions makes them flexible and versatile to any use case that a business could think of. It is a very powerful use of real-world data to drive business decisions that can have an unimaginable impact on optimizing performance, cost, engagement, or any other metrics crucial for the business use case.
Specifically for retail analytics companies, the biggest use case lies with optimizing their expansion plans. The traditional methods of approaching the problem of expansion with physical location scouting and deploying field teams are based more on judgment rather than logic. Hence, there is no way to validate the decisions made through these practices. Moreover, there is no way to predict the performance and revenue of the new stores as there is no data backing these decisions. Modern machine learning techniques used in location-based intelligence make this process more reliable and predictive.
Today, real-world data is readily available and we have developed sophisticated technologies to transform this data into a structured and usable format. The location data from various sources, once collected and transformed, becomes a gold mine to draw insights into the physical expansion of retail businesses. The brief idea behind optimizing the expansion plan for a business is to identify the best existing stores and find new locations using real-world data with similar conditions: socio-economic, demographic, level of infrastructure, population density, and many more. When businesses eliminate the judgment factor, and back site selection by real-world data, they can even predict the revenue and performance for each site they consider with minimum scope of error.
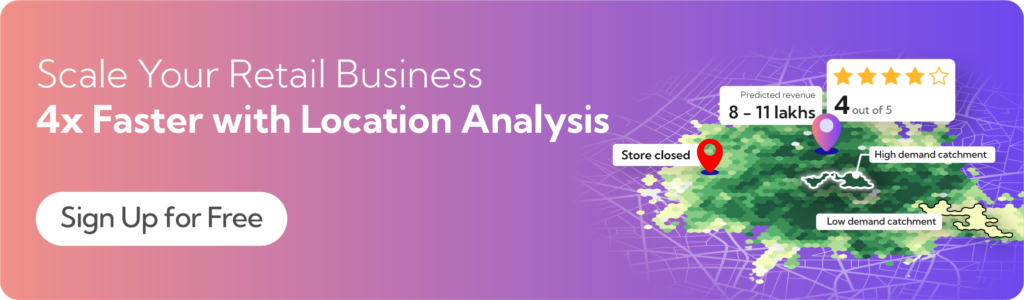
Lenskart Problem Statement:
Lenskart wants to expand its presence with new retail stores across the country and needs hyperlocal intelligence to finalize site selection. Some of the specific requirements are:
1. Revenue prediction
2. Cost indicators especially rental information
3. Profit prediction
4. Information on Cannibalization from new locations
5. Footfall prediction
Before moving on to the solution, here’s a small overview of Lenskart and the cause it caters to, and last year’s performance statistics.
About Lenskart
Peyush Bansal’s Lenskart is revolutionizing the eyewear industry in India. The retail brand was born to solve a very pressing issue in society that had been sidelined for many years until Lenskart was founded. Their commitment to customer satisfaction with constant innovation has led to tremendous growth and support from those who believe in their cause.
1/3rd of the country’s population needs vision correction but does not have access to proper eye care and glasses. With over 15 million blind people across the country, India can be named the blind capital of the world.
With this cause in mind, Peyush Bansal along with co-founders Amit Chaudhary and Sumeet Kapahi founded Lenskart.
The aim was to eliminate the retailers by setting up high-quality manufacturing capabilities and supplying directly to consumers across India. This model would help Lenskart reduce costs and maintain high-quality standards for its eyewear products. The in-house robotic lens manufacturing and assembling ensure 100% precision and top quality control.
Offering the best quality products at affordable prices helped Lenskart to be positioned among the top 3 optical businesses in India. From servicing 30 customers per day to more than 3000, the retail major has come a long way.
Lenskart is a growing business that has shown outstanding growth over the years and that can be attributed to the technology innovations and data-backed decision-making. Looking at the figures for the fiscal year 2021, Lenskart has shown a 4.6x growth in its profits.
As it should, the major revenue source for the business is the sale of products via multiple retail channels including physical stores and online channels. The product sale generated INR 855.7 crore during FY21 as compared to INR 851.2 crore in FY20.
The physical outlets form an important element of the retail presence of Lenskart for the following reasons:
- The eye-checkup facility attracts more footfall and boosts in-store sale
- The overall experience enabled by the try and buy set-up
- Serves as a destination for those seeking ideas and inspiration around their personal style
- Fast turn around on product delivery
- Knowledge and expertise of medical and sales staff
- Better customer engagement and satisfaction resulting in loyalty
With an aim of opening around a good chunk of stores in near future, Lenskart aims to create a robust network of physical outlets and as it is in its nature, wants to deploy logic and use real-world data to finalize locations in a way that maximizes revenue.
Before we move on to the solution and hyperlocal intelligence that GeoIQ uses to solve this problem, let us have a look at how the traditional market expansion works and what are its limitations and challenges
Traditional Physical Expansion Process for Retail Businesses
For many years now, retail businesses have been relying on their teams that are physically present at their branch offices, or ground forces that help them scout favorable locations for their new stores. Even though these methods consider certain parameters to shortlist favorable locations, the decision largely depends on individual judgments and suffers from human biases.
Even with the manual site selection approach, there are various factors that are considered while shortlisting locations, such as:
- Demographics and the population size of the target audience
- Existing Competition and how they are performing
- Infrastructure
- Other businesses present nearby
- Foot traffic, and more
But even after considering all these factors and shortlisting the sites, the final selection of the locations does not hold any logical ground. The reason is that there is no data to validate the choices.
There are various limitations and challenges to this traditional method:
- The decisions made by business heads could be influenced by individual experience and preferences
- Individual judgments could overpower the facts and decisions could be impacted
- The ground teams scouting for locations might not have required clarity on the kind of product, its positioning, and target audience
- There is no real-world data to validate site choices and final decisions
- There is no real-world data to predict revenue and profit from new locations
These limitations could result in lost opportunities. There is a high probability that the site that was discarded could have performed much better than the site that was selected. Moreover, there is no data again to validate such lost opportunities.
Hence, it is very critical today, especially with the availability of massive volumes of data, that some kind of intelligence be considered while expanding retail presence with new stores. In the next section, we will see how Lenskart has benefited by adopting GeoIQ’s No-code machine learning model for hyperlocal intelligence to set up their new stores.
GeoIQ’s No-Code ML Model
GeoIQ helps identify location-based patterns in a set of data and predict look-alike behaviors across geographies. The real-world data from over 600 trusted data sources is taken into account to provide hyperlocal intelligence for 2000+ relevant attributes. Our Machine Learning model helps Lenskart with its expansion plan. Here’s how the solution works for this use case:
Data Collection:
Lenskart provides the performance data of their existing stores along with details like store size, company-owned/franchise-owned stores, opening date, closing date, staff size, the number of ophthalmologists, and others.

The image showcases existing Lenskart store locations. The green spots are high-performing locations and the red ones are non-performing.
Data Cleaning and Preprocessing:
The data collected from Lenskart goes through automated preprocessing and restructuring to account for the following:
- Some of the stores were relatively new and not matured in terms of sales
- The correct potential was not recorded due to the impact of covid
- Less control on information from franchise-owned franchise-operated stores as compared to company-owned company-operated stores
- De-seasonalizing sales figures
The transformed data is then ready to be ingested into the machine learning model.
Model Selection:
A regression model is used to predict monthly revenue for each prospective location. This is how the model works:
- The model takes the existing store performance data as input, the fields essentially are the store’s address and the revenue generated per month
- Creating custom catchments around existing locations ranging from 200m to 5km radii to study attributes present in these areas
- The model maps the relevant attributes (from a list of more than 2000 identified attributes) with the performing and non-performing stores respectively
- Now we have a set of identifiers that would score a new location based on the presence of attributes relevant to the performing or non-performing stores
- The model would also predict the revenue that could be generated for each location depending upon the presence of identified GeoIQ attributes, plus Lenskart’s inputs on rental cost and property size
- Last but not the least, it predicts footfall which directly translates into revenue
GeoIQ offers 2000+ attributes sourced from 600+ varied data sources across categories. Have a look at our data catalog here: https://catalog.geoiq.io/in
Training and Testing:
The model is trained on the sample data, i.e., the data of existing Lenskart stores. Once the model starts predicting results, it is tested on another data set and the error margins are identified. The idea here is to have a good split of data across cities and not just Tier 1 and metropolitans. This avoids bias while predicting cities beyond these categories.

The image showcases predicted locations for new Lenskart stores. The green spots are favorable locations and the red ones are non-favorable.
The Impact
The model is able to predict the revenue that would be generated over the coming months by a newly opened store. Now the impact of choosing all the right locations when combined together can be massive.
- Revenue, when maximized at the store level, would combine to exponentially increase the overall annual revenue
- The opportunity lost due to faulty decisions would be minimized
- The effect of cannibalization from new stores could be minimized
Here are visualization charts that depict the revenue trends validated by the GeoIQ ML model for newly opened Lenskart stores. Graph 1 shows the average revenue projections (low, mid, and high revenue stores) for stores that were opened in the month of January. The actual data for the months of January to March validated the model predictions for each store.
Similarly, Graph 2 shows the revenue projections for the stores that were opened in February, and again the actual revenue numbers of each store validated the model results.
So what does this information convey?
Now that the model is successfully trained and tested for predicting the revenue of each store, it can identify the look-alike locations and tag them as high or low-performing sites. The model essentially gives each new location a score, the higher the score, the better the performance of that site.
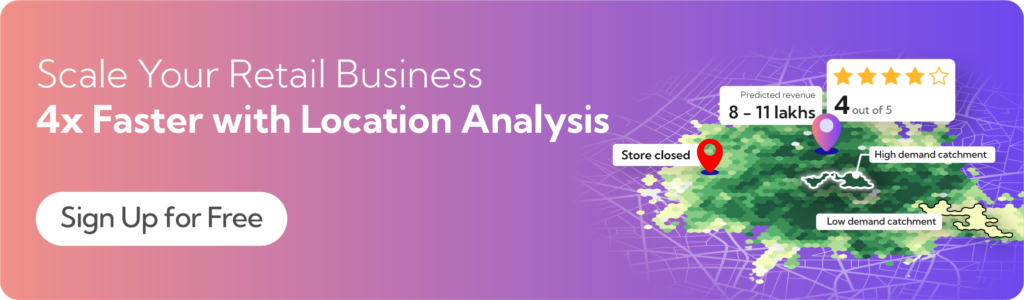
With this system in place, the Lenskart site selection process is now simplified to a level whereby just looking at the model score for a location, officials can decide if it is an ideal location for their upcoming store.