The answer lies in location data and AI-based prediction models. While placing products across stores, intelligent merch-mix and demand prediction answers help you optimise your inventory distribution across stores. This trickles down to improved sales, reduction in dead stock, and inter-store inventory transfers.
Your sales data only tells part of the story. Most inventory analysis and sales reports don’t take into account the influence of external factors, which can range from weather and local events to macroeconomic factors and market conditions. GeoIQ has built-in external data sets like points of interest in a catchment, civic infrastructure, and demographic data, that provide context, help improve feature interpretability, and reinforce model performance with a comprehensive viewpoint.
Let’s look into two examples of location data-backed merch mix decisions that added to the store’s revenue success and inventory management.
Use Case 1:
Constraint: One of our clients wanted to limit their premium product range exposure to 120 stores.
The brand ran an experiment to validate the results by creating a test-control set across three categories of stores based on the revenue generated. Category A is the high revenue generating stores, Category B is the medium revenue generating stores, and Category C is the low revenue generating stores. Within each category, we further split them into stores with high, medium, or low affinity for premium products. We arrived at this categorisation based on the location characteristics of existing stores where premium products have done well in the past.
The test sets were stores where the premium products were placed and control sets, where the premium products were not placed.
To validate the hypothesis of whether GeoIQ was giving the right set of stores, the brand’s team did an A/B testing for these products and placed the premium products in all 120 stores.
Count of store code | Increase in sales per store | |
---|---|---|
Cat A | 41 | |
High Affinity for premium products | 15 | 1.5X |
Medium Affinity for premium products | 17 | 1.13X |
Low Affinity for premium products | 9 | X |
Cat B | 33 | |
High Affinity for premium products | 5 | 2Y |
Medium Affinity for premium products | 9 | 1.25Y |
Low Affinity for premium products | 19 | Y |
Cat C | 16 | |
High Affinity for premium products | 5 | No visible increase |
Medium Affinity for premium products | 5 | No visible increase |
Low Affinity for premium products | 6 | No visible increase |
Inference:
Within high and medium-revenue-generating stores, there was a visible increase in the sales of the overall store by placing premium products. The stores with proven high affinity for premium products showed 2 times (in the medium revenue category), and 1.5 times (in the high revenue category) more sales as compared to the stores with lower affinity for premium products.
The Result: The set of stores recommended by GeoIQ achieved 45% more sales.
After this experiment, GeoIQ was asked to recommend stores from the whole universe of stores for the new product launch. Making this a conclusive exercise for the brand and building confidence.
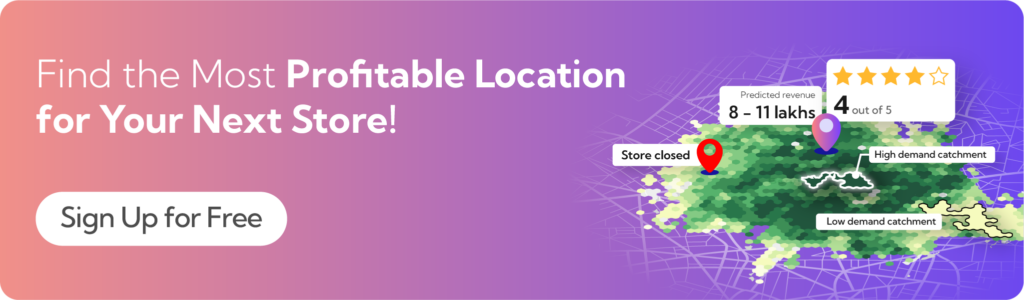
Use Case 2:
Constraint: A premium furniture and mattress brand wanted to identify stores to place high-value mattresses
A premium furniture and mattress brand aimed to pinpoint the ideal store locations to showcase their premium products.
Our analysis revealed an intriguing insight: while factors like income levels and spending preferences are the obvious indicators to gauge the appetite for premium products, the lack of granular data and rapidly evolving urban landscapes make it challenging to identify such markets. This highlights the need for indicators that can subtly capture these trends.
For instance, our study found that localities with a high concentration of dental clinics and extracurricular activity centers often reflect a spending behavior that goes beyond essentials—signaling a focus on wellness and a well-rounded lifestyle.
Conclusion:
The results of these use cases underscore the transformative impact of location data and AI-powered prediction models in merchandise placement. By leveraging GeoIQ’s insights, brands can not only optimise inventory allocation but also achieve significant improvements in sales and operational efficiency.
In the first case, the thoughtful selection of stores based on revenue categories and affinity for premium products resulted in a 45% increase in sales—proving the value of precise, data-backed decisions. The second case further illustrates how sometimes unconventional indicators can reveal hidden market potential for high-value products.
GeoIQ’s ability to integrate diverse external datasets—such as demographic patterns, Points-of-Interest, and local infrastructure—ensures that brands gain a holistic perspective on their catchment areas. This enables them to make well-informed decisions that resonate with market demand while minimizing risks like dead stock or over-distribution.