Like any other business, for a fitness brand growth is dependent on being accessible to the right audience on the right street across multiple cities. Apart from this. there are multiple concerns for a fitness chain to address while opening new fitness centers, some of which are:
- Identifying the total addressable market (smaller micro-markets) within a city, frequented by their ideal TG.
- A fitness center cannot serve beyond capacity, hence understanding demand and balancing for cannibalization and unmet capacity while placing new facilities.
- Predict revenue at each location to ensure success.
For a major fitness brand in India, GeoIQ became their partner in growth, using advanced machine-learning algorithms, to predict revenue and demand at multiple sites.
In this case study, we will expound on our strategy and procedure to derive an equitable solution for the client. We divided our solution suites into two parts,
- A custom algorithm identifying the exact audience of the fitness brand
- A self-serve product used by the business development team, state expansion heads, revenue team, etc.
This case study will also cover the following topics:
- The expansion challenges faced by the leadership of the national fitness chain.
- GeoIQ’s process & methodology
- How we calculated the maximum number of fitness centers they could operate profitably before reaching saturation.
- Develop a comprehensive insight into our solutions for the national fitness brand.
- Understand its existing demand
- Pinpoint the locations with significant demand
- Understand their revenue projections in a given geography
- Leverage our data to negotiate favorable rental terms
Problem Statement
- The fitness chain wanted to identify profitable site locations in cities nationwide for expansion.
- Assess market saturation within a pin code to avoid cannibalization
- Reduce breakeven time at each center
Challenges of a National Fitness Chain
- Revenue Vs Rentals: Being profitable is a function of revenue vs rentals. While the rentals are known, revenue can never be predicted in the very human-centric decision process.
- Cannibalization and Churn: The basis of fitness centers is real estate, a finite resource within cities. There are only so many centers a fitness chain can open before it starts to cannibalize its existing stores. Fitness centers also have to deal with churn. According to a report by the IHRSA (2020), fitness centers lose more than 50% of their members yearly. To arrest this loss in revenue, the fitness center offers passes allowing fitness enthusiasts to access their entry-level gyms.
- Market Saturation: There is a fixed time when fitness enthusiasts use a center. This can be in the morning or evening, but the number of users in the interim reduces drastically. Thus, it is easier for a fitness center to reach a saturation point inside a pincode, necessitating expanding to new locations. The solution to this question is not a function of the total demand in a location, but where the demand is located.
- Capital Expenditure Cost: With the high CapEx cost associated with opening a fitness center, their breakeven time must be reduced while increasing their profitability.
Methodology
GeoIQ is a leader in precise & granular location data that is available for 300 cities across India including Metro, Tier 1, Tier 2 & beyond. Our data is available down to a granularity of 100m catchments. This data includes population, retail, infrastructure, healthcare & many more, and feeds into our pre-built Machine Learning models for omnichannel businesses.
GeoIQ offers a tailored suite of pre-built AI models, each model is meticulously fine-tuned to meet the specific needs of each client. The GeoIQ machine-learning algorithm is a robust location-intelligence model validated by back-testing unknown center locations for the fitness chain against the actual performance of these centers.
We worked with the brand on their offline and online data on user behavior. Following this, we defined the model we would use to build our algorithm. The AI model tries to figure out reasons for good & bad performing locations and explains them using location variables. GeoIQ then determined the features/variables positively correlated with positive revenue & vice versa.
We programmed the variables into the GeoIQ model, which, through iterative learning, assigned a score for the various parameters responsible for the success of a fitness center.
These variables include,
- Population Density
- Footfall
- Growth Trends
- Presence of Complementary and Competitor Brands
- Retail Landscape
- Accessibility Quotient
GeoIQ uses the weighted score of these variables to determine if a selected location will be profitable for the client. Based on the presence of these categories, we could determine the Total Addressable Market for the fitness chain in any given location.
Solution
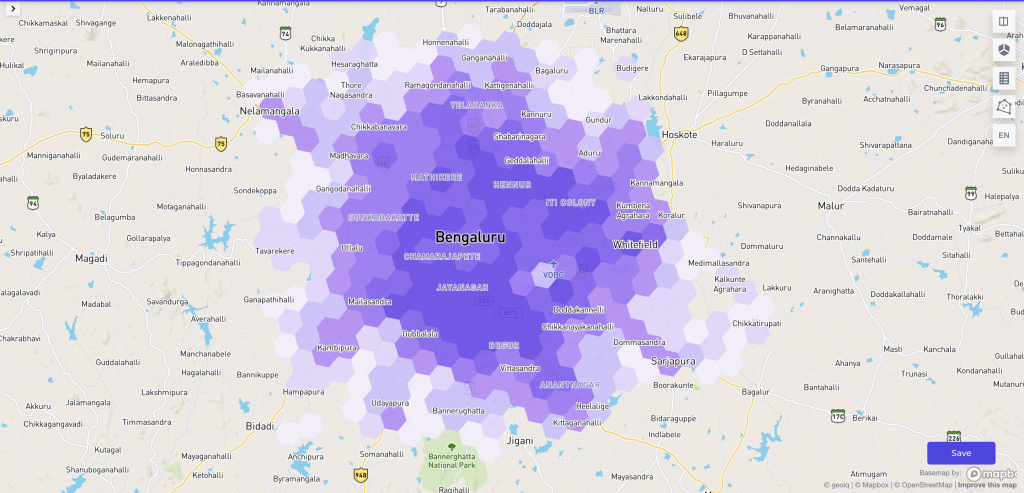
Example of a TAM Map: Utilizing the TAM map above, the fitness chain’s leadership can strategically categorize city locations based on their preference for a fitness center. The hexagons, which are bright green, represent the high presence of the target audience, with the ones in pale white representing the low presence of the ideal customer for the respective brand.
Data-backed Decision Making: Data enables clients to eliminate gut-based decision-making when expanding into a new location. GeoIQ employs verifiable metrics to determine the success of a site.
- The GeoIQ model enables business expansion managers to get AI-powered locations in any cities planned for expansion. This helps them prioritize markets before proceeding towards property scouting.
- During the property scouting process, each prospective site is validated with a potential revenue score which helps in closing a property quickly in a fast-moving market. Gone are the days of losing shortlisted properties because of slow gut-based decisions.
Demand Estimation and Market Penetration: The RetailIQ model helps the business heads estimate the average demand in a given location. Based on this report, the fitness chain can direct its marketing efforts to increase sales in the selected location. The RetailIQ model also helps the fitness chain estimate their penetration rate in the given geography, helping them identify their total possible revenue. This allowed the fitness chain to build new fitness centers and contributed to their expansion.
Revenue Projection: RetailIQ’s machine learning model empowers the fitness chain to project the total revenue potential within a specific location. The model generates a score for each geographic location the fitness chain is considering for expansion. The fitness chain uses this information to identify the ideal areas for expansion.
Understanding Revenue-Rental: Revenue-Rental ratio determines the payback period for brands. It helps give a relative indication of the future potential while helping them create expansion plans.
Inferences
Dynamics of Variables: We discovered that the variable that makes a location profitable requires a multifaceted approach. For example, through our analysis, we were able to identify the importance of apartment complexes to determine the profitability of a fitness center. But, in the HSR layout of Bangalore, there is a real shortage to the number of apartment complexes, and yet the fitness centers in this location are profitable. This is because of the presence of other complementary variables that improve the profitability of the location.
User Clusters: After analyzing the data, we pinpointed clusters of fitness enthusiasts across the city, each with varying population densities. Taking Bangalore as an example, we found that the entire Total Addressable Market (TAM) of fitness enthusiasts was concentrated in just 30 clusters, covering only 20% of the city. Focusing on these major areas could effectively encompass the entirety of their expansion in Bangalore.
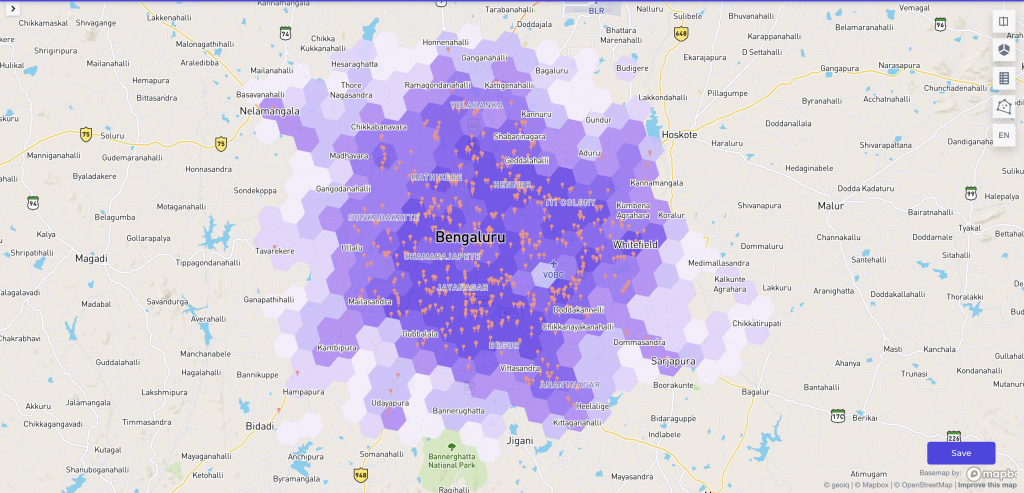
In this representative map, notice the presence of competitors and complementary brands next to areas with a large presence of the fitness center’s target audience.
Presence of Complimentary and Competitor Brands: Plotting the locations of complementary and competitor brands and overlaying it with the TAM demand map of a city helps the brand determine the areas with a large presence of the fitness center’s target audience. Since the TG of the complementary and competitor brands share similar variables, the fitness chain will be able to identify the profitable locations to start a new fitness center.
Impact
Retail Insights: GeoIQ’s solutions empower retail chain business development teams to make informed, data-backed decisions, enhancing both efficiency and effectiveness. The insights derived from GeoIQ not only facilitate the growth of retail chains but also illuminate the untapped potential within a market. GeoIQ acts as a catalyst, removing growth constraints and propelling retail chains into an accelerated expansion phase.
Visualizing Success: GeoIQ’s machine learning unveils geo-patterns, empowering retail clients to:
- Slash underperforming stores by 50%
- Trim payback periods by 45%
- Accelerate finding profitable locations by 4x
Tailored-made Solutions for Retail Expansion: The model accurately forecasts the prospective revenue of a newly established store in the upcoming months.
The cumulative impact of strategically selecting optimal locations is substantial:
- Store-level revenue maximization contributes to an exponential boost in annual revenue
- Minimizes the losses incurred by flawed decisions
- Mitigates the risk of cannibalization from new stores.
What Is RetailIQ
RetailIQ is a cutting-edge AI solution revolutionizing the retail sector by seamlessly integrating location data. It is a powerful tool for site recommendation and analysis, helping brands maximize revenue, minimize store closure risk, identify market potential, and refine target audiences down to street-level granularity. With custom models fine-tuned for individual brands and complementary brand analysis, RetailIQ surfaces tailored insights. Beyond recommendations, it offers comprehensive site analysis, giving retailers a competitive edge in profitable expansion and predictable growth through informed data-driven decisions.