A Brief About the Kitchen Appliance Chain
In this case study, we are considering the expansion requirement for a company that has leveraged its traditionally strong presence in the kitchenware industry to reimagine itself as a kitchen appliance company catering to a new audience.
After being listed on the NSE, the brand leveraged its traditionally strong presence in the kitchenware industry to reimagine itself as a kitchen appliances major.
It is a big jump in brand positioning where the need for exclusive stores becomes crucial to substantiate this shift. Reimagining the brand also paves a growth path for their stakeholders.
When entering into the new categories, the brand also had to reimagine multiple unknowns, i.e., identify new addressable markets and the new target audience and its characteristics. To successfully break into this new avatar, the traditional teams and the brand realized that their existing approach to expansion would not suffice.
A new data-led approach was needed to crack the successful offline network of their new exclusive stores. The situation is not very different from a D2C brand looking ahead to opening its first offline store.
The Problem Statement
- Identifying the new target audience and its characteristics, suited better for their new offerings
- Identify new markets to expand where this target audience shops
The kitchen appliance major requires a pragmatic system to categorize its audience and capture a slice of the $3 billion Indian kitchen appliance market.
The transformational pivot undertaken by the kitchen appliance chain required a reimagining of its potential target audience. The brand had to develop new strategies to identify and connect with its new target group. The existing teams of these brands lack the nuances involved with mapping the interests of the new target audience.
The brand’s business team wanted GeoIQ’s help to understand how implementing data-backed location strategies will help accelerate the brand’s expansion. They wanted to identify their core demographics better and provide granular street-level recommendations for expansion.
Identify where the TG is present and that will indicate where to expand.
GeoIQ and the Magic of Machine Learning Models
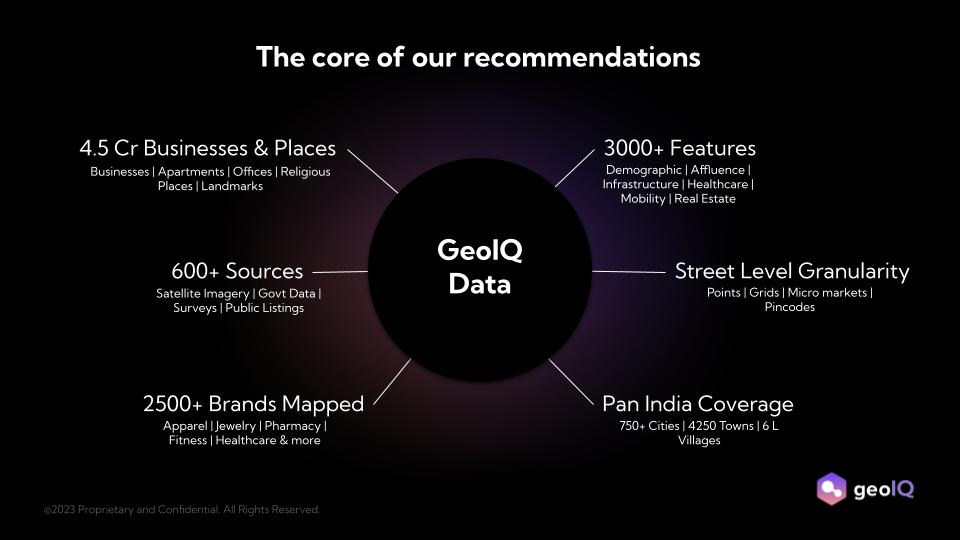
The question was simple.
How can the brand growth hack its business while generating a massive footprint and increasing its brand presence?
To help GeoIQ solve its problem, the brand provided sales data from the 40+ stores in Bangalore.
Using this data, we classified the stores into two categories, good and non-performing stores. We based the categorization on the sales revenue number as defined by the business logic of the executives at this kitchen appliance brand.
After plotting the location of the good and bad stores, we started identifying the underlying reasons why good stores do well and why non-performing stores do poorly.
What did our AI models discover?
The successful stores are not located on the high street but are concentrated in neighborhood markets.
And that was the Eureka moment for the brand.
We began to classify the Points Of Interest (POIs) around the 40+ stores in Bangalore considering a range of around one kilometer from the store location. This helped us determine the exact mix of POIs responsible for the success or non-performance of a store.
What is a Point of Interest?
Point of Interest data defines a physical location represented by its Latitude and Longitude. Examples of POI include; Banks, Hospitals, Schools, etc. Simply put, a POI is any location visible on a map, both physical and virtual (Google Maps)
Why are Points of Interest (POIs) important?
They provide essential reference points for projects, business strategies, and decision-making, offering valuable data points. This data helps in mapping competition in a trade area, understanding TG characteristics and spending patterns, footfall trends, and more. E.g. How far is the nearest competitor, how close is a metro station, etc
Identifying the Target Group of the Kitchen Appliance Chain
Target Groups are important because they tend to behave in predictable patterns helping data scientists generate granular street-level maps that are beneficial to the brand.
For example, the ideal customer of the kitchen appliance company could be a person who shops at bakeries and grocery stores, and they wouldn’t be living on or near the high street.
This precise understanding of the behavior of the target customer helps us refine our model and define other locations with matching characteristics.
Some of the POIs identified from our investigation into the TG of kitchen appliance brands are the following,
Positive indicators
- Pet stores
- Labs
- Grocery Shops
Negative indicators
- High-end apparel stores
- Shopping complex
The pattern of the presence of POIs around the brand’s good and non-performing stores helped us identify the following about its target audience,
- They are part of a new or growing family
- A family who is conscious about their health
- The presence of families who are budget-friendly
- Families who do not live on/near the high street
From our investigations, these positive and negative correlations are indicative of the presence of a neighborhood market.
This validated the inference by our AI model which predicted the presence of a neighborhood market in the vicinity of the successful store.
How Does This Brand Use This Intelligence?
Street-level accurate data can potentially transform a business’s growth trajectory.
The reimagined kitchen appliance brand wanted to focus its expansion efforts in Southern India. But, in just two quarters they are now considering the North Indian market.
This is a result of the business teams leveraging the insights from GeoIQ to determine if the properties they have considered for expansion are a good fit for their business. Thus, reducing the number of non-performing stores and increasing their net revenue.
Traditionally, the head of expansion at a retail brand would direct their BD teams into certain pockets to scout for properties to open new exclusive stores based on prevailing property market sentiments.
The BD team would then return with a list of properties best suited for expansion based on the brand’s defined success criteria. However, these listings would be tainted with human biases and influenced by the broker’s preferences.
Expanding businesses also tend to employ guesswork to determine the profitability of any location. This process introduces errors into the decision-making process.
GeoIQ developed a solution-oriented custom AI model that provides the business development team at the brand with a comprehensive site report.
In this site report, the AI model grades properties using GeoIQs’s custom scores.
This grading allows the business expansion head to have an overview of the recommended location on the same scale. Chances are that a BD team member would identify a property that meets all the criteria for opening a successful exclusive store. GeoIQ models would uncover hidden problems that would turn this potentially successful location into a non-performing store.
GeoIQ’s models remove the uncertainty associated with determining a profitable location by applying data-backed analysis. This makes the step of identifying a successful location objective rather than subjective.
Impact of GeoIQ’s Retail Analytics Solution
Retail Insights: GeoIQ’s solutions empower retail chain business development teams to make informed, data-backed decisions, enhancing both efficiency and effectiveness. The insights derived from GeoIQ not only facilitate the growth of retail chains but also illuminate the untapped potential within a market. GeoIQ acts as a catalyst, removing growth constraints and propelling retail chains into an accelerated expansion phase.
Visualizing Success: GeoIQ’s machine learning unveils geo-patterns, empowering retail clients to:
- Slash underperforming stores by 50%
- Trim payback periods by 45%
- Accelerate finding profitable locations by 4x
Tailor-made Solutions for Retail Expansion: The model accurately forecasts the prospective revenue of a newly established store in the upcoming months.
The cumulative impact of strategically selecting optimal locations is substantial:
- Store-level revenue maximization contributes to an exponential boost in annual revenue
- Minimizes the losses incurred by flawed decisions
- Mitigates the risk of cannibalization from new stores.
What Is RetailIQ
RetailIQ is a cutting-edge AI solution revolutionizing offline expansion for the retail section. It is built on top of extensive location data and brand data to give street-level answers to expansion problems.
RetailIQ is a powerful tool providing site recommendations to maximize success at the store level and minimize the risk of closure. It also helps in identifying the total addressable market, competition analysis, a better understanding of your target audience traits and their presence, detailed site analysis, and reports, all adding up to a successful expansion strategy.