In the competitive world of retail, success hinges on choosing the right locations for new stores. A prime location can differentiate between a bustling store and a shuttered storefront. To tackle these challenges, predictive analytics becomes a game-changer that empowers retailers to make data-driven decisions, minimize risks, and maximize success.
This article delves into how predictive analytics can transform retail expansion strategies, ensuring that new stores meet the location characteristics associated with success and significantly reduce the chances of store closure.
Retail Use Cases of Predictive Analytics in Retail
Predictive analytics leverages advanced data analysis techniques to forecast future trends and behaviors. By analyzing vast amounts of data, predictive models can provide actionable insights that drive strategic decision-making. In retail, predictive analytics transforms location selection from a gamble into a calculated strategy, allowing retailers to identify high-potential sites with precision.
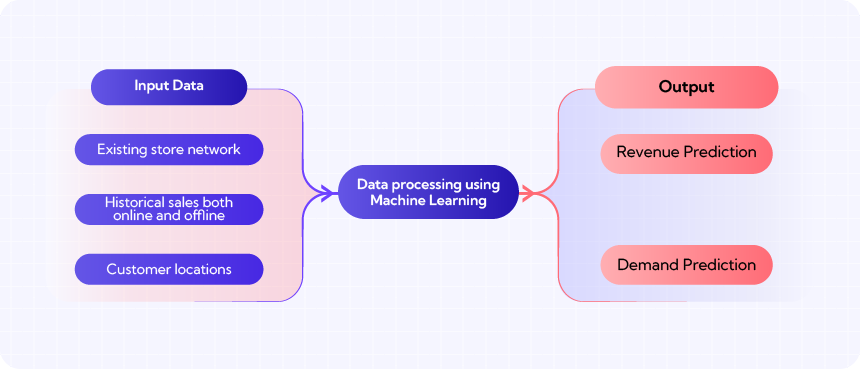
Here are some challenges that could be solved with predictive analytics atop location data.
Revenue Prediction
Predictive analytics in retail allows businesses to forecast potential revenue for new store locations by analyzing various factors contributing to sales performance. Machine learning models can assess the characteristics of a possible location, such as demographic profiles, average income levels, foot traffic patterns, and proximity to competitors. By incorporating historical sales data from existing stores and these location variables, the model can predict how much revenue a new store will likely generate at a given site.
For example, a retailer looking to open a new store can use predictive analytics to estimate that a location in a high-traffic shopping district with a young, affluent demographic might generate higher revenue compared to a location in a less populated area.
Risk of Store Closure
Predictive models analyze data such as past performance of stores in similar areas and specific location characteristics that could lead to store closures.
For instance, predictive analytics might reveal that a prospective location has a high risk of store closure due to unfavorable factors such as low foot traffic, inadequate visibility, or insufficient parking facilities, among other reasons. By identifying these risks based on historical data and location-specific attributes, retailers can avoid sites with high closure probabilities and choose more favorable locations, ensuring a more stable and profitable expansion.
Demand Prediction
Predictive models analyze historical sales data, economic indicators, and local events to forecast demand accurately. Moreover, analyzing trade area characteristics and the presence of competing and complimentary brands among other factors, can help identify the total addressable market for a brand in a particular geography.
Profitable Site Selection
Choosing the right location for a new store is critical, and predictive analytics transforms this decision-making process by providing data-driven insights. Instead of relying solely on intuition, retailers can use predictive models to analyze factors such as demographic data, local consumer spending habits, nearby brands and POIs, footfall patterns, trade area and total addressable market, demand gap, and more.
For example, predictive analytics might reveal that a location near a university with high student foot traffic and limited retail competition is likely to be highly profitable. By identifying such high-potential sites, retailers can strategically expand their footprint, ensuring that new stores are positioned in locations with the highest revenue potential.
Growth Trends
By analyzing online data, historic sales data, consumer data, market reports, infrastructure development trends, emerging tech hubs and residential areas, and more, predictive models can highlight emerging neighborhoods or areas with increasing consumer demand.
For instance, a retailer might discover that a previously overlooked suburb is experiencing rapid population growth and an influx of young families, indicating a promising location for a new store. By recognizing these growth trends, retailers can proactively establish a presence in burgeoning areas, capturing market share and driving sustainable business growth before competitors do.
Future Trends in Predictive Analytics for Retail
Retail tech is constantly evolving; especially because the retail sector is extremely agile towards adapting to new technologies and innovative solutions to sell more and better. Some of the trends that one can foresee transforming the retail sector in the near future are:
- Emerging Technologies: Advances in AI and ML will make predictive models more accurate and adaptive, providing deeper insights into consumer behavior and allowing for real-time data integration through IoT.
- Evolving Consumer Preferences: Predictive analytics will help retailers stay ahead of shifting consumer trends by analyzing social media, online reviews, and purchase behaviors, enabling them to adapt their product offerings swiftly.
- Accurate Footfall Prediction: Improved data collection and analysis techniques will enhance footfall forecasting accuracy by considering variables such as local events, weather conditions, and consumer movement patterns, helping retailers optimize store layouts and staffing.
- Hyper-Personalization: Predictive analytics will enable hyper-personalized shopping experiences by analyzing individual consumer preferences and behaviors, allowing retailers to offer tailored recommendations and promotions.
- Operational Efficiency: Enhanced predictive models will optimize supply chain and inventory management, reducing waste and ensuring that high-demand products are always available.
- Omni-channel Integration: Predictive analytics will unify data across all sales channels, providing a seamless shopping experience and consistent customer insights for better decision-making.
Conclusion
Predictive analytics is revolutionizing retail expansion by providing retailers with the insights needed to choose optimal store locations. By leveraging data to predict demand, analyze location characteristics, and minimize risks, retailers can open new stores with confidence and achieve long-term success. As the retail landscape continues to evolve, predictive analytics will remain a vital tool for driving strategic growth, ensuring profitability, and maintaining a competitive edge.