A renowned name in the premium dessert category sought data-driven insights to identify high-revenue areas for its next phase of expansion.
With a growing footprint and increasing demand for its natural and artisanal ice creams, the brand aimed to leverage GeoIQ’s location intelligence to shortlist profitable locations for its expansion that would drive higher revenue for both online and offline channels.
About the Client
Our client is a leader in the premium ice cream industry, known for its innovative use of real fruits and natural ingredients. The brand’s journey began with a simple yet groundbreaking idea: to create ice creams that not only carried the essence of fruit flavours but also incorporated real fruits themselves.
With a legacy spanning decades, today, the client operates 169 outlets across India, delighting millions of customers with its curated range of fruit-based and traditional ice creams.
The Challenge
- Identifying high-revenue zones that cater to both online and offline sales.
The Outcome
By leveraging GeoIQ’s location data and machine learning model, our client gained actionable insights into ideal locations across India, enabling them to strategically target profitable locations for expansion.
Methodology
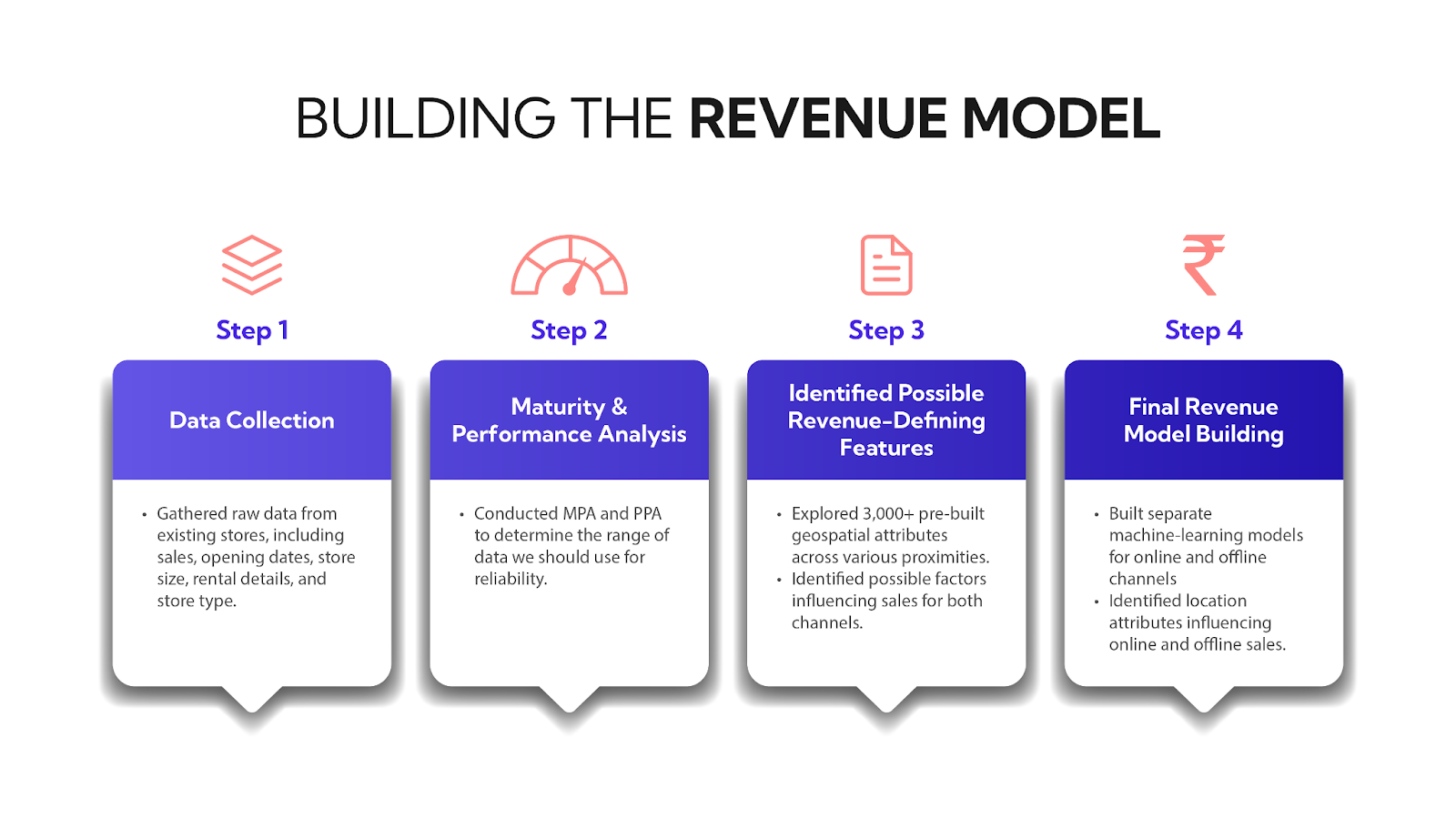
—
To support our client’s expansion goals, we focused on identifying the key location attributes that influence the sales of their existing stores – both online and offline.
By identifying and analysing these attributes, we were able to recommend new locations based on various defining factors, which will be detailed later.
To achieve this, we built separate machine learning models, ie, revenue models for online and offline channels that leveraged historical store data to learn and identify successful patterns.
These models are designed to analyse a wide range of location attributes and run simulations on potential locations to recommend high-potential sites for expansion.
Here’s a step-by-step explanation of how we built our revenue model:
Step 1: Store data collection
We began the process by collecting various data points from the last 2 years, from 169 stores across 47 cities.
The collected data includes:
Store Specifications:
- Store Code
- Store Name
- Store Ownership (COCO/FOFO)
- Store Opening Date
- Store Type (Mall, Tech Park, High Street)
- Store Size
- Seating Capacity
- Closing Date (If any store closed in last 2 years)
- Reason for Store Closure
Store Sales Data:
- Store Revenue (Offline & Online)
- Number of In-Store Receipts
- Number of Online Transaction Receipts
We then sanity checked these data to ensure that the data we fed to the model was reliable and accurate.
Step 2: Conducting performance and maturity period analysis
To understand the timeframe we should take to build the final revenue model we have conducted,
Maturity Period Analysis – to identify the time it takes for a store’s sales to stabilize after opening. On average, we found that the maturity period for the stores is 6 months.
Performance Period Analysis – to understand the consistency of the performance throughout the year.
Conducting these analyses helps us determine the most reliable timeframe for building a reliable revenue model that accounts for both stabilized sales and seasonal performance patterns.
Step 3: Data exploration
With our initial revenue model correlating these attributes with existing store data, we were able to understand the possible reasons behind a store’s successful sales for both online and offline channels.
Step 4: Building the revenue model & Selecting attributes
For our final revenue model, we used data from 130 stores for training, including average revenue from the past 12 months, while the remaining stores were used for testing the model’s accuracy.
With the said data, we built a separate revenue model for online and offline channels to ensure the GeoIQ-recommended location can experience high revenue from both channels.
These models were then trained and used to analyse different locations across the target cities to identify areas with high revenue potential.
The model then analysed the factors contributing to overall sales and prioritized the key features, out of 3,000, that have a direct impact on sales.
Below are the key revenue-defining attributes our model has narrowed down to:
Insights
Key factors influencing revenue for existing stores: Offline
i) Location attributes that impact offline sales positively:
Location Attribute | Impact |
Footfall in restaurant and dessert stores | 28% |
Presence of a high number of clinics | 12% |
Store Size (in sq. ft) | 11% |
Overall footfall | 8% |
Presence of branded electronics | 8% |
ii) Location attributes that impact offline sales negatively:
Location Attribute | Impact |
Presence of Food delivery restaurants | 11% |
Presence of grocery stores | 10% |
Presence of branded pharmacies | 5% |
Presence of medium-value apartments | 4% |
Key factors influencing revenue for existing stores: Online
i) Location attributes that impact online sales positively:
Location Attribute | Impact |
Presence of residential apartments and houses | 28% |
Presence of high end branded salons | 17% |
Internet speed | 14% |
Presence of high end gyms | 12% |
Income above 20 LPA | 10% |
Population within a specific socioeconomic bracket | 8% |
Store Size (in sq. ft) | 6% |
ii) Location attributes that impact offline sales negatively:
Location Attribute | Impact |
Presence of food retailers | 5% |
These identified attributes helped us understand the key factors that drive sales and revenue across both online and offline channels.
With these insights, we can effectively start shortlisting high-potential locations for the client, ensuring that new stores are positioned in locations with the best chance of success!